The Future of Driving changes dramatically with the advent of technology converging to introduce humankind to a very new concept of convenience and productivity: self-driving cars. Both fully autonomous and advanced driver-assistance-capable vehicles rely on a wide variety of different AI techniques to operate safely and efficiently in complex, real-world settings. Mastering the range of these techniques will not only illuminate the issue of how self-driving cars function but also what the future of transportation holds.
1. Machine Learning: Backbone of Autonomous vehicles Driving
Machine learning is a subset of AI and forms the backbone of most self-driving car systems so that they can learn from data and improve performance over time without explicit programming. Various forms of machine learning are in use today. Few of the most critical techniques include:
a. Supervised Learning
Training a model in supervised learning means it’s trained on a labeled dataset. That is to say, for every input in the training dataset, its desired output is known. Such is the approach followed for autonomous vehicles in order to classify and identify objects in their environment, identification of pedestrians, other vehicles, and other obstacles like traffic signs. Image classification is one of the more active uses with this pattern; therefore, algorithms can teach the system, after being trained using thousands of images with objects labeled in these, to recognize, differentiate, and detect, in real time, any such object or obstacle.
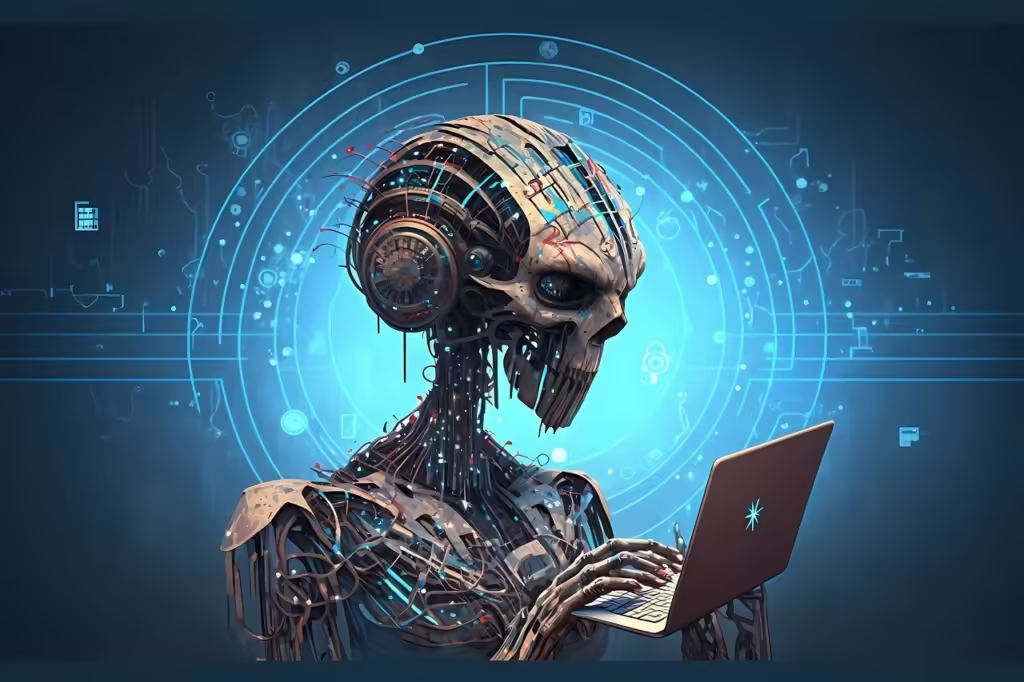
Reinforcement Learning
In reinforcement learning, an agent learns through actions taken in an environment with the objective of maximizing cumulative reward. It finds most useful applications in scenarios that are complex, where the optimal actions are not predefined. In self-driving cars, reinforcement learning will be applied to optimize decision-making and maneuvering tasks like changing lanes, merging, and going through intersections. The vehicle learns from trial and error, fine-tuning its approach through simulated or real-world driving experiences.
The Future of Driving Unsupervised Learning
Unsupervised learning gives a clear insight into how unlabeled data are analyzed for the detection of patterns and structures. This will be very helpful in clustering similar driving conditions, patterns, and behaviors, which may be helpful in enhancing the car’s adaptiveness in different scenarios and situations.
2. Computer Vision: Perception is Key
Computer vision is another cornerstone of self-driving technology, which empowers the vehicle to interpret and understand visual information from the world around them. Advanced cameras, Light Detection and Ranging-LIDAR-and radar provide the requisite data that their computer vision algorithms will use to create a detailed depiction of the vehicle’s surroundings.
a. Object Detection and Recognition vehicles
These will have the effect of enabling instantaneous classification and identification of an object in a real-time self-driving car using a deep learning architecture known as convolutional neural networks. CNN has a role to play in the processing of pixel data, generalizing to recognize an object even when its condition is different-say, due to a difference in light or weather circumstances.
b. Pixel-wise classification
Semantic segmentation is the technique used for categorizing each pixel of the image in a meaningful way and making distinctions between roads, sidewalks, vehicles, and pedestrians. This level of detail will enable the self-driving car to make an informed decision about path and behavior in a dynamically changing environment.
3. Sensor Fusion: Putting It All Together
To cope with the diverse set of situations at hand, autonomous vehicles employ the use of **sensor fusion**, software input from many different sensors combined into one view of the world.
a. Complementary Strengths
Each one of these sensors has its strong and weak points: while LIDAR can give good estimations of distance but may be sloppy with textureless surfaces, cameras capture abundant information visually but are vulnerable to lighting changes. By fusing information from multiple sources, sensor fusion enables the car to perceive its environment more robustly and more accurately in a complementary manner; hence, improving safety.
b. Real-Time Processing vehicles
This fused data, in turn, is fed into the AI algorithms in real time, enabling the vehicle to respond instantly: to a leading vehicle braking suddenly, for example, or to pedestrians crossing at an intersection. The efficiency of these algorithms significantly impinges upon general performance and safety in self-driving technology.
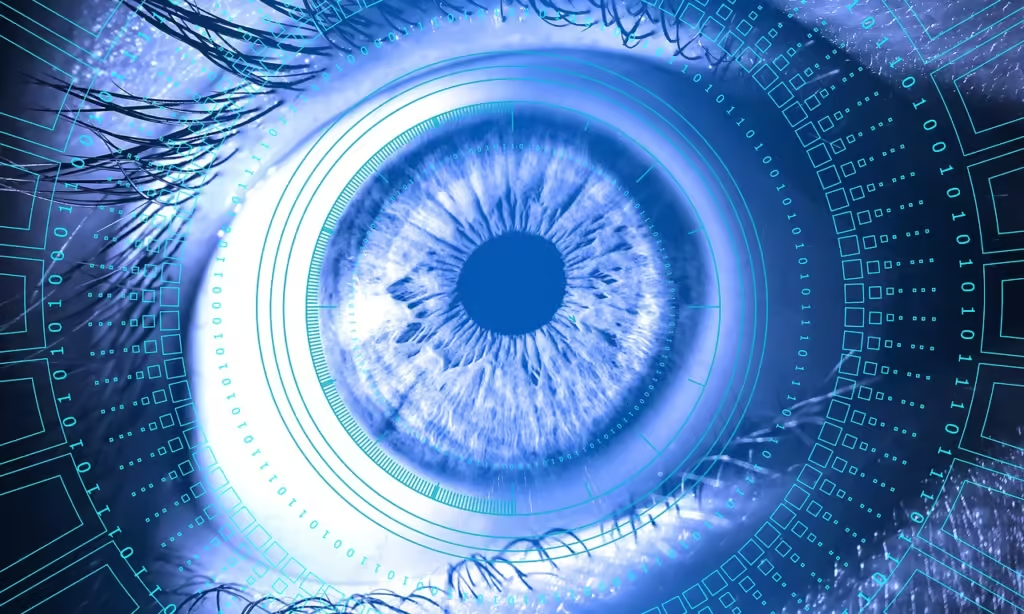
4. Localization and Mapping: Knowing Where You Are
For a self-driving car, for navigation to be fully proper, there is an essential need for always being absolutely sure of the exact position. This, in many ways, requires advanced techniques for localization and the environmental mapping in detail.
The Future of Driving vehicles a. High-Definition Maps
HD mapping is a subject involving the creation of detailed digital versions of on-road environment features such as lane markings, road signs, and other features and landmarks. In most cases, LIDAR would be used to create these maps, and real-time driving data updates them constantly.
b. Simultaneous Localization and Mapping
SLAM is a technology that allows one vehicle to build a map while at the same time keeping its position in an unknown environment. It is very important for self-driving cars since it helps them get through areas which might be new or dynamic and where no maps may have existed previously.
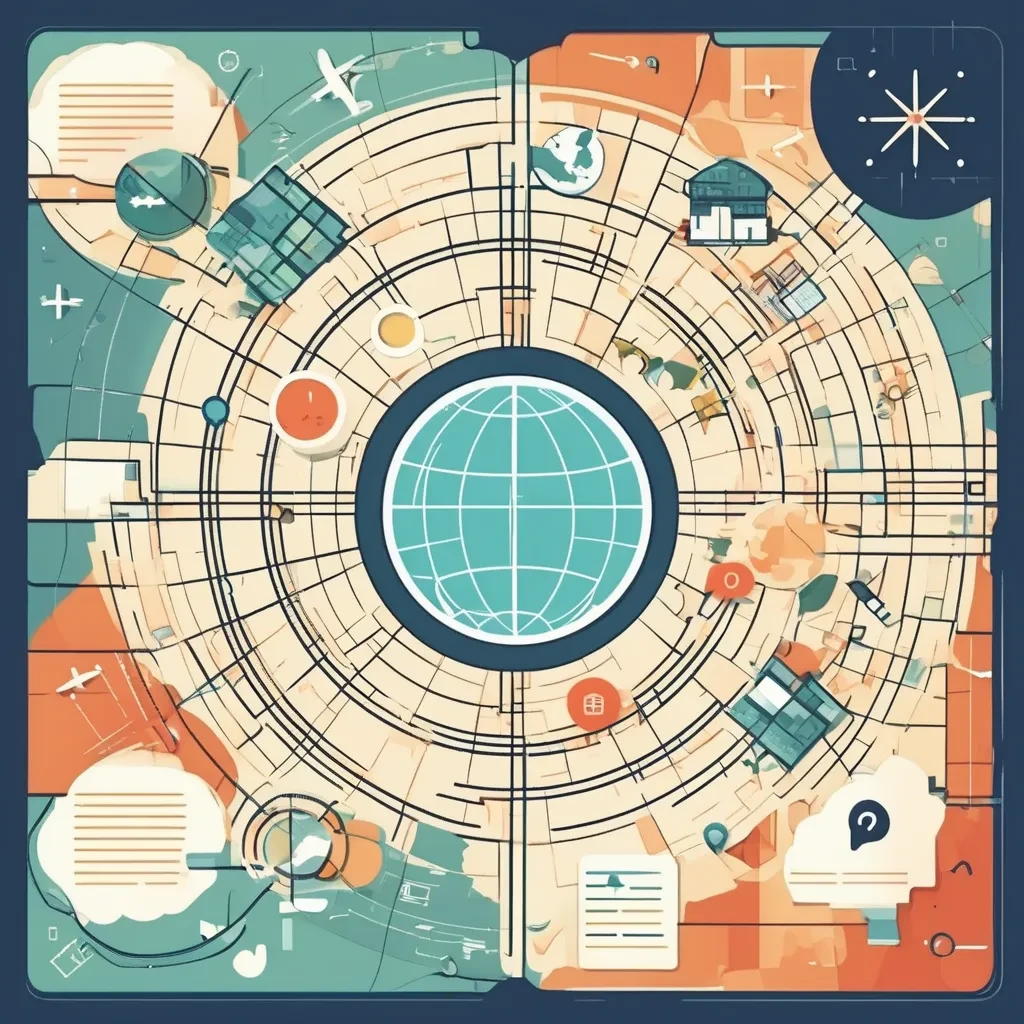
5. Path Planning and Control: Making Safe Decisions
Once the autonomous vehicle has perceived the scene and established its position, it needs to make decisions on how to safely reach its desired destination. It follows that the appropriate path planning and control systems must be robust.
a. Path Planning Algorithms
In path planning, the purpose is to compute an optimal road that the vehicle shall take according to some factors like road conditions, traffic, and dynamics of the vehicle. Therefore, this usually entails different algorithms in the computation of safe and efficient paths, such as A* or Rapidly-exploring Random Tree.
Control systems should execute the devised trajectory regarding vehicle speed, acceleration, and steering. For safety reasons, control systems need to respond promptly to changes in the driving environment.
6. Ethical and Social Considerations
The real-world deployment of technology of autonomous driving raises significant ethical and social issues: How should the vehicles act in emergency situations? Ensuring data privacy and addressing regulatory challenges will be paramount concerns that will require careful consideration.
Conclusion
Self-driving cars, being one of the most exciting uses of AI, will make navigation safe in the complex driving arena of the future with its power drawn from the realms of machine learning, computer vision, sensor fusion, and sophisticated localization and planning techniques. And while much work is yet to be done-regulatory frameworks, addressing of public concerns, among others-the bedrock of work done in these AI techniques forms a base for times when self-driving cars might just redefine the way people travel. As technology advances, clears up, and improves, the possibilities for innovation in the automation of transport systems become increasingly clear, brighter, and assure safer, efficient, and user-friendly travel.